How To Say Univariate
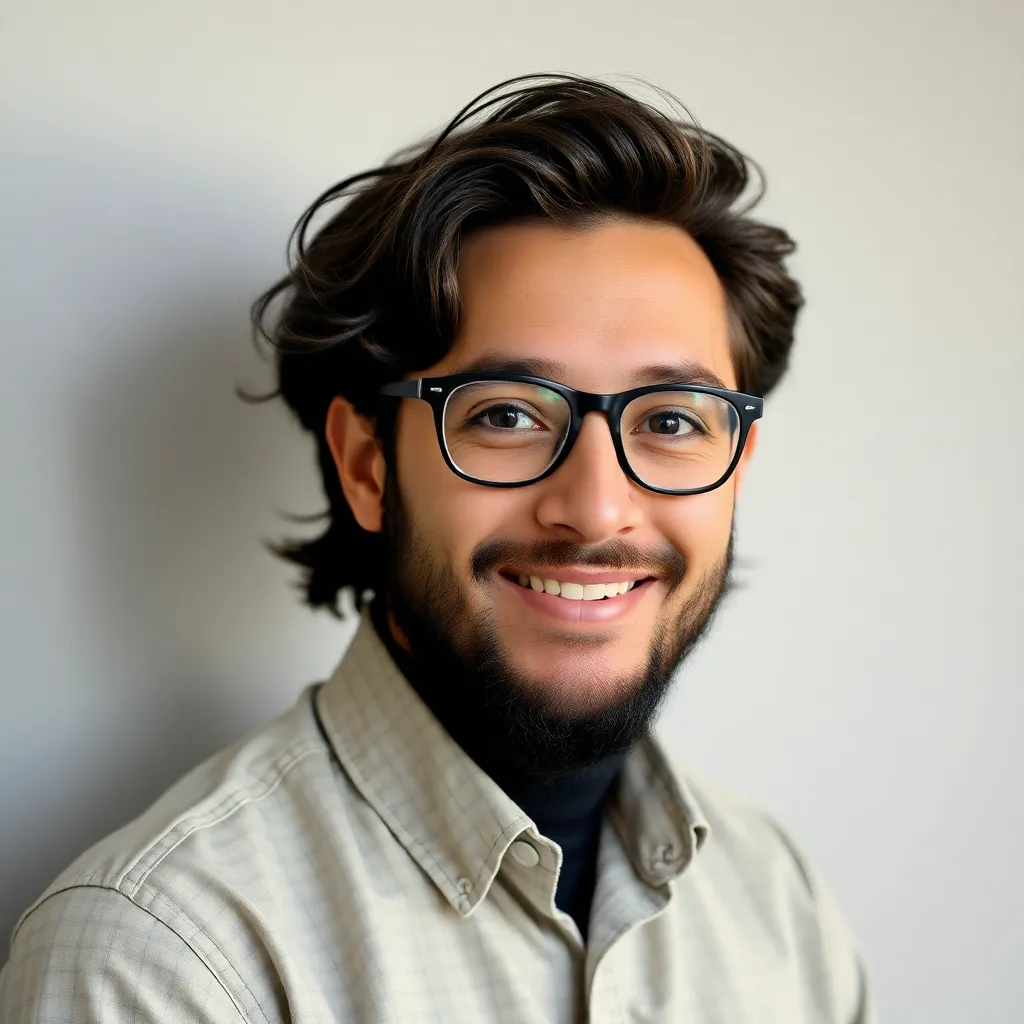
adminse
Apr 06, 2025 · 10 min read
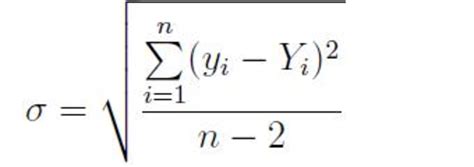
Table of Contents
How to Say "Univariate": Understanding and Applying This Statistical Term
What makes understanding "univariate" so crucial for data analysis?
Mastering univariate analysis is the cornerstone of effective data interpretation and unlocks a world of insights.
Editor’s Note: This comprehensive guide to understanding and applying "univariate" in data analysis was published today.
Why "Univariate" Matters
The term "univariate" might seem intimidating at first glance, but it's a fundamental concept in statistics. Understanding it is crucial for anyone working with data, whether in academia, business, or any field involving data analysis. Univariate analysis, the process of analyzing a single variable, forms the bedrock upon which more complex multivariate analyses are built. Without a solid grasp of univariate methods, interpreting more sophisticated analyses becomes significantly more challenging. It's the foundational step in understanding the distribution, central tendency, and variability of your data – key elements for drawing accurate and meaningful conclusions. Its applications span diverse fields, from understanding customer demographics in marketing to analyzing clinical trial results in medicine, and predicting stock prices in finance. The ability to effectively describe and summarize single variables is indispensable for making informed decisions based on data.
Overview of the Article
This article will explore the core meaning of "univariate," providing a comprehensive explanation of its usage within the context of statistical analysis. We will delve into the various techniques used in univariate analysis, demonstrate their practical applications through real-world examples, and address common misconceptions. Readers will gain a thorough understanding of univariate analysis, its importance, and how to effectively interpret its results. The article will also discuss the limitations of univariate analysis and when it's appropriate to move beyond single-variable analysis to more complex methods.
Research and Effort Behind the Insights
The information presented in this article is based on extensive research, drawing upon established statistical literature, textbooks, and online resources. The explanations are grounded in established statistical principles and aim to provide a clear and accessible understanding of univariate analysis for a broad audience. We have carefully selected examples to illustrate the practical application of the concepts discussed.
Key Takeaways
Key Concept | Description |
---|---|
Univariate Analysis | The analysis of a single variable. |
Descriptive Statistics | Summarizing data using measures like mean, median, mode, variance, and standard deviation. |
Inferential Statistics | Drawing conclusions about a population based on a sample, using techniques like hypothesis testing. |
Data Visualization | Graphical representations of data, such as histograms, box plots, and frequency distributions. |
Identifying Data Distributions | Determining if data follows a normal, skewed, or other distribution. |
Limitations of Univariate Analysis | Cannot assess relationships between variables; only describes individual variables. |
Smooth Transition to Core Discussion
Let's delve into the key aspects of univariate analysis, starting with its definition and moving on to explore the various methods and techniques employed.
Exploring the Key Aspects of Univariate Analysis
-
Defining Univariate: Univariate, at its core, means "single variable." In statistical analysis, it refers to the examination of a single variable at a time, independent of other variables. This contrasts with bivariate (two variables) and multivariate (more than two variables) analyses.
-
Descriptive Statistics in Univariate Analysis: The primary goal of univariate analysis is often to describe the characteristics of the single variable under scrutiny. This involves calculating descriptive statistics. These statistics provide a concise summary of the data's central tendency, dispersion, and shape. Key descriptive statistics include:
-
Measures of Central Tendency: These describe the "center" of the data. The most common are the mean (average), median (middle value), and mode (most frequent value). The choice of which measure to use depends on the data's distribution and potential outliers.
-
Measures of Dispersion: These describe the spread or variability of the data. Key measures include the range (difference between the highest and lowest values), variance (average of squared deviations from the mean), and standard deviation (square root of the variance). The standard deviation is particularly useful as it provides a measure of variability in the same units as the original data.
-
Shape of the Distribution: Univariate analysis also helps in understanding the shape of the data distribution. This can be assessed visually using histograms or box plots, and can be described as normal (bell-shaped), skewed (asymmetrical), or uniform (evenly distributed). Understanding the shape is critical for choosing appropriate statistical tests and interpreting results.
-
-
Inferential Statistics in Univariate Analysis: While descriptive statistics summarize the observed data, inferential statistics allow us to make inferences about a larger population based on a sample from that population. In a univariate context, this involves techniques like:
-
Hypothesis Testing: This involves testing a specific hypothesis about the population parameter (e.g., the population mean). Common univariate hypothesis tests include the t-test (for comparing a sample mean to a known population mean or comparing the means of two independent groups) and the z-test (similar to the t-test but used when the population standard deviation is known).
-
Confidence Intervals: These provide a range of values within which the true population parameter is likely to fall with a certain level of confidence. For example, a 95% confidence interval for the population mean indicates that there's a 95% probability that the true population mean lies within that range.
-
-
Data Visualization in Univariate Analysis: Visualizing the data is an essential part of univariate analysis. Graphs and charts help in quickly understanding the distribution, central tendency, and variability of the data. Common visualizations used include:
-
Histograms: These show the frequency distribution of the data, providing a visual representation of its shape.
-
Box Plots: These display the median, quartiles, and potential outliers of the data, providing a concise summary of its central tendency and dispersion.
-
Frequency Distributions: These tables show the frequency (count) of each unique value in the dataset.
-
Closing Insights
Univariate analysis, despite its apparent simplicity, forms a critical foundation for all data analysis. Its ability to provide a clear and concise summary of a single variable's characteristics is essential for understanding the data before proceeding to more complex analyses. By mastering the techniques of descriptive and inferential statistics, and utilizing appropriate visualizations, researchers and analysts can extract valuable insights from their data. The ability to identify the shape of the distribution and interpret key statistics is fundamental for informed decision-making in any field utilizing data.
Exploring the Connection Between "Data Cleaning" and "Univariate Analysis"
Data cleaning is inextricably linked to univariate analysis. Before conducting any univariate analysis, it's crucial to clean the data. This involves identifying and handling missing values, outliers, and inconsistencies. Univariate analysis plays a critical role in this process. For example, histograms and box plots can help identify outliers, while frequency distributions can reveal inconsistencies or errors in data entry. Without proper data cleaning, the results of univariate analysis can be misleading or inaccurate. Methods for handling missing data—such as imputation (filling in missing values based on other data points) or exclusion (removing cases with missing values)—are often informed by the univariate distribution of the variable. Similarly, dealing with outliers might involve transformations (e.g., logarithmic transformations to reduce the influence of extreme values) which are often assessed within the context of the univariate distribution. Therefore, data cleaning and univariate analysis are interdependent steps in the broader data analysis workflow.
Further Analysis of "Data Cleaning"
Data cleaning is not merely a preliminary step; it's an iterative process that often requires revisiting and refining as the analysis progresses. Identifying potential errors or inconsistencies requires careful examination of the univariate distributions of each variable. For example, if a variable is supposed to represent age, values outside of a plausible range (e.g., negative ages) are clear indicators of errors. Similarly, unexpected spikes or gaps in the frequency distribution might indicate problems with data entry or coding. Dealing with these issues can involve various techniques, including:
-
Identifying and Handling Missing Data: Missing data can bias results and should be addressed. Methods include imputation (replacing missing values with estimated values), deletion (removing cases with missing data), or using specialized statistical methods designed for incomplete data. The choice depends on the extent of missingness and the nature of the data.
-
Identifying and Handling Outliers: Outliers are data points that are significantly different from the rest of the data. They can be caused by errors or represent legitimate extreme values. Techniques for handling outliers include transformation, winsorizing (replacing extreme values with less extreme values), or robust statistical methods that are less sensitive to outliers.
-
Checking for Data Consistency: It's crucial to check for inconsistencies in the data, such as misspellings, incorrect formatting, or duplicate entries. This often involves cross-checking against other data sources or using data validation tools.
-
Data Transformation: Data transformation can improve the normality of a distribution, address heteroscedasticity (unequal variances), or reduce the influence of outliers. Techniques include logarithmic, square root, or Box-Cox transformations. The appropriateness of each transformation is often guided by the initial univariate distribution of the variable.
FAQ Section
-
Q: What is the difference between univariate, bivariate, and multivariate analysis? A: Univariate analysis examines a single variable, bivariate analysis examines two variables, and multivariate analysis examines more than two variables.
-
Q: Why is univariate analysis important? A: It forms the basis of more complex analyses. It helps understand individual variable characteristics and identify potential issues in the data before further analysis.
-
Q: What are the common methods used in univariate analysis? A: Descriptive statistics (mean, median, mode, standard deviation, etc.), inferential statistics (hypothesis testing, confidence intervals), and data visualization (histograms, box plots).
-
Q: How do I choose the right type of graph for univariate analysis? A: Histograms are useful for visualizing the distribution of continuous data, while bar charts are suitable for categorical data. Box plots effectively display the spread and central tendency of data.
-
Q: What are the limitations of univariate analysis? A: It cannot assess relationships between variables. It only describes the characteristics of individual variables.
-
Q: When should I move beyond univariate analysis? A: When you want to understand relationships between variables, predict outcomes, or use more complex modeling techniques, multivariate analysis is necessary.
Practical Tips
-
Always start with data cleaning: Address missing values, outliers, and inconsistencies before any analysis.
-
Use appropriate descriptive statistics: Choose the measures of central tendency and dispersion that are most suitable for the data's distribution.
-
Visualize your data: Histograms, box plots, and frequency distributions help in understanding the data's characteristics.
-
Conduct hypothesis tests if appropriate: Use inferential statistics to make inferences about the population based on your sample.
-
Interpret results carefully: Understand the limitations of univariate analysis and avoid overinterpreting findings.
-
Document your analysis: Keep a record of the methods used and the results obtained for reproducibility and clarity.
-
Consider data transformations: If the data doesn’t meet assumptions of some statistical tests, consider appropriate transformations.
-
Use software for analysis: Statistical software packages like R, SPSS, or SAS can automate calculations and produce visualizations.
Final Conclusion
Univariate analysis, while seemingly a simple statistical method, plays a fundamental role in the data analysis process. It provides a necessary foundation for understanding individual variables, identifying data quality issues, and laying the groundwork for more advanced multivariate analysis. By mastering the concepts and techniques discussed in this article, you will be better equipped to extract valuable insights from your data and make more informed decisions. The ability to effectively describe, summarize, and interpret univariate data is a crucial skill for anyone working with data, regardless of their specific field. Further exploration into advanced statistical methods builds directly upon this fundamental understanding. Remember that the power of univariate analysis lies in its simplicity and its capacity to provide a solid base for more complex analyses.
Latest Posts
Latest Posts
-
How To Say Cinderace
Apr 07, 2025
-
How To Say Naples In Spanish
Apr 07, 2025
-
How To Say All The Best For New College
Apr 07, 2025
-
How To Say Happy Birthday In Russia
Apr 07, 2025
-
How To Say Noely
Apr 07, 2025
Related Post
Thank you for visiting our website which covers about How To Say Univariate . We hope the information provided has been useful to you. Feel free to contact us if you have any questions or need further assistance. See you next time and don't miss to bookmark.